08 August 2024
- RSIS
- Publication
- RSIS Publications
- Deciphering the Language of Internet Memes, its Use in Disinformation and Detection via AI
SYNOPSIS
In the current socio-digital milieu, internet memes, “a unit of cultural transmission”, play an increasing role in online discourse and interactions. Traditionally channels for humour, social commentary, and cultural expression, internet memes also have a dark side to them. They can cause real harm through their capacity to spread negative sentiments, misinformation, disinformation, hate and violence. Detection of memes is hence critical to prevent harmful effects on society, but it poses significant challenges. Some projects focusing on the detection and classification of memes via the use of AI are discussed.
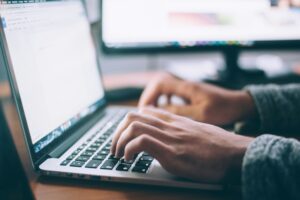
COMMENTARY
The 2019 Christchurch terrorist attack serves as a stark example of how internet memes have been used to spread hate and inspire violence. Upon the attacker’s incitement, certain online communities created and spread hundreds of memes that celebrated the killings and idolised the attacker as a cult-like religious figure which were then used to create “fan” merchandise for sale. Other communities have created and shared memes as a way to mock the impact of the 9/11 attack every year on the anniversary of the deadly attacks in New York.
Memes are becoming a reflection of our contemporary culture, an easily reproducible “unit of cultural transmission”. According to reports, 55 per cent of internet users aged 13 to 35 engage in weekly meme sharing, while 30 per cent do so on a daily basis.
While most memes are meant for cultural expression and humour, it has emerged that memes also have a dark side to them as they can cause real harm beyond the confines of the digital world through their capacity to incorporate images and videos that are excised from their original context to spread negative sentiments, misinformation, disinformation, hate, fear and violence.
Memes in Disinformation
Memes have also come to play a key role in information warfare. The ongoing Russia-Ukraine War has observably become an arena in which memes have been deployed to spread disinformation online. TikTok has come to be used as a medium for the propagation of memes, with “WarTok” (a portmanteau of “TikTok” and “war”) being a space in which people have shared fake, AI-generated videos of the war.
Misinformation and disinformation, whether spread through memes or other relevant pathways, have the potential to erode trust in institutions, undermine democratic processes, and foster social division. The identification of these types of harmful content, whether they take the form of images, videos or memes, is therefore an important endeavour, as it would help to prevent or at the least mitigate their harmful effects on society.
Challenges in Detecting Harmful Memes
There have since been numerous projects launched with the aim of automating the process of harmful meme detection at scale, owing to the sheer volume of memes that are shared every second across all forms of social media. These projects aim to develop AI-powered algorithms to analyse, assess, and classify potentially harmful memes in a more efficient, automated manner.
However, a significant challenge that faces developers of such algorithms stems from the inherently multi-modal nature of memes. Many memes often comprise images, accompanied by a text caption; they include a textual modality, and an image modality. Problems arise when AI algorithms designed to detect harmful content in memes are not well-equipped to handle multiple modalities at once.
A meme might include text that would otherwise be considered benign or innocuous when the text is considered in isolation. However, this very same text could take on new, more malicious meaning when considered in conjunction with the image it accompanies. An example of such a meme can be viewed here. The textual modality of the meme must be considered in conjunction with the image modality of the meme for a more well-informed, accurate assessment on whether a particular meme is potentially harmful or not.
Research Efforts in Harmful Meme Detection
Some of the projects currently being undertaken at the Macquarie University School of Computing in Australia are examples of ongoing efforts to develop better harmful meme detection algorithms that account for the multi-modalities inherent in meme content. These projects aim at different aspects of harmful meme detection and include (i) identifying misinformation in memes, (ii) detecting misinformation in historical multimodal memes, and (iii) detecting harmful memes.
One of the challenges faced is that current analysis tools and models lack the ability to account for contextual information that might heavily define or skew the meaning of a meme.
One of the projects aims to develop an AI algorithm that captures the context of an entire post, accounting for textual content, image content, and synthesising the two to provide an assessment of whether a post might contain misinformation.
Another project in a yet to be published study focuses on developing an algorithm to analyse the historical posts of a particular user in order to assess whether they might be an active vector for spreading misinformation. Reinforcement learning-based models are employed to siphon out irrelevant posts from relevant ones, with measures put in place to identify certain important keywords, i.e., “depopulation”, “propaganda”, “Bill Gates”, etc., that might suggest that misinformation is being spread.
A third project aims to develop a prompt-based approach to identifying harmful or hateful memes that might target certain communities and/or individuals.
The last project aims to improve cross-modal (language and image) alignment across all the other three projects. The Cross-Modal Aligner algorithm developed for this project generates a set of questions and answers as prompts that would in turn produce better, more accurate textual descriptions of images that are fed into it. These textual descriptions can then be deployed in other harmful meme detection algorithms to improve their reliability and results.
These projects are still under development and more efforts will continue to be required to design new methods for robust detection of these memes.
Ways Forward
Like deepfakes, memes pose a potentially serious pathway for misinformation, disinformation, hate speech, and other harmful content to be propagated en masse throughout society. It would thus be in the interests of governments and regulatory bodies to work with academic research groups and other entities who are actively developing algorithms and technologies to decipher the language of memes and detect harmful ones.
Funding and support initiatives like the recently announced Centre for Advanced Technologies in Online Safety (CATOS) could expedite their efforts to improve their AI models in order to better account for the multi-modal nature of memes, and to collate together larger and more comprehensive datasets to train their algorithms.
Furthermore, more open lines of communication and exchange of data between research teams and regulatory bodies should be fostered. Research teams may continuously and regularly update government regulatory bodies on the latest forms of harmful memes that have come into circulation and vogue, so that counter-messaging operations may be planned and deployed to stem the negative effects of such memes on their respective societies.
About the Authors
Usman Naseem is Lecturer at the Macquarie University School of Computing, Australia. Asha Hemrajani and Tan E-Reng are Senior Fellow and Research Analyst, respectively, at the Centre of Excellence for National Security (CENS), S. Rajaratnam School of International Studies (RSIS), Nanyang Technological University (NTU), Singapore.
SYNOPSIS
In the current socio-digital milieu, internet memes, “a unit of cultural transmission”, play an increasing role in online discourse and interactions. Traditionally channels for humour, social commentary, and cultural expression, internet memes also have a dark side to them. They can cause real harm through their capacity to spread negative sentiments, misinformation, disinformation, hate and violence. Detection of memes is hence critical to prevent harmful effects on society, but it poses significant challenges. Some projects focusing on the detection and classification of memes via the use of AI are discussed.
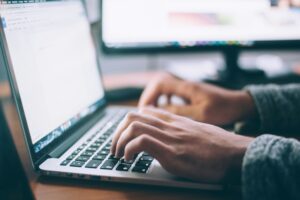
COMMENTARY
The 2019 Christchurch terrorist attack serves as a stark example of how internet memes have been used to spread hate and inspire violence. Upon the attacker’s incitement, certain online communities created and spread hundreds of memes that celebrated the killings and idolised the attacker as a cult-like religious figure which were then used to create “fan” merchandise for sale. Other communities have created and shared memes as a way to mock the impact of the 9/11 attack every year on the anniversary of the deadly attacks in New York.
Memes are becoming a reflection of our contemporary culture, an easily reproducible “unit of cultural transmission”. According to reports, 55 per cent of internet users aged 13 to 35 engage in weekly meme sharing, while 30 per cent do so on a daily basis.
While most memes are meant for cultural expression and humour, it has emerged that memes also have a dark side to them as they can cause real harm beyond the confines of the digital world through their capacity to incorporate images and videos that are excised from their original context to spread negative sentiments, misinformation, disinformation, hate, fear and violence.
Memes in Disinformation
Memes have also come to play a key role in information warfare. The ongoing Russia-Ukraine War has observably become an arena in which memes have been deployed to spread disinformation online. TikTok has come to be used as a medium for the propagation of memes, with “WarTok” (a portmanteau of “TikTok” and “war”) being a space in which people have shared fake, AI-generated videos of the war.
Misinformation and disinformation, whether spread through memes or other relevant pathways, have the potential to erode trust in institutions, undermine democratic processes, and foster social division. The identification of these types of harmful content, whether they take the form of images, videos or memes, is therefore an important endeavour, as it would help to prevent or at the least mitigate their harmful effects on society.
Challenges in Detecting Harmful Memes
There have since been numerous projects launched with the aim of automating the process of harmful meme detection at scale, owing to the sheer volume of memes that are shared every second across all forms of social media. These projects aim to develop AI-powered algorithms to analyse, assess, and classify potentially harmful memes in a more efficient, automated manner.
However, a significant challenge that faces developers of such algorithms stems from the inherently multi-modal nature of memes. Many memes often comprise images, accompanied by a text caption; they include a textual modality, and an image modality. Problems arise when AI algorithms designed to detect harmful content in memes are not well-equipped to handle multiple modalities at once.
A meme might include text that would otherwise be considered benign or innocuous when the text is considered in isolation. However, this very same text could take on new, more malicious meaning when considered in conjunction with the image it accompanies. An example of such a meme can be viewed here. The textual modality of the meme must be considered in conjunction with the image modality of the meme for a more well-informed, accurate assessment on whether a particular meme is potentially harmful or not.
Research Efforts in Harmful Meme Detection
Some of the projects currently being undertaken at the Macquarie University School of Computing in Australia are examples of ongoing efforts to develop better harmful meme detection algorithms that account for the multi-modalities inherent in meme content. These projects aim at different aspects of harmful meme detection and include (i) identifying misinformation in memes, (ii) detecting misinformation in historical multimodal memes, and (iii) detecting harmful memes.
One of the challenges faced is that current analysis tools and models lack the ability to account for contextual information that might heavily define or skew the meaning of a meme.
One of the projects aims to develop an AI algorithm that captures the context of an entire post, accounting for textual content, image content, and synthesising the two to provide an assessment of whether a post might contain misinformation.
Another project in a yet to be published study focuses on developing an algorithm to analyse the historical posts of a particular user in order to assess whether they might be an active vector for spreading misinformation. Reinforcement learning-based models are employed to siphon out irrelevant posts from relevant ones, with measures put in place to identify certain important keywords, i.e., “depopulation”, “propaganda”, “Bill Gates”, etc., that might suggest that misinformation is being spread.
A third project aims to develop a prompt-based approach to identifying harmful or hateful memes that might target certain communities and/or individuals.
The last project aims to improve cross-modal (language and image) alignment across all the other three projects. The Cross-Modal Aligner algorithm developed for this project generates a set of questions and answers as prompts that would in turn produce better, more accurate textual descriptions of images that are fed into it. These textual descriptions can then be deployed in other harmful meme detection algorithms to improve their reliability and results.
These projects are still under development and more efforts will continue to be required to design new methods for robust detection of these memes.
Ways Forward
Like deepfakes, memes pose a potentially serious pathway for misinformation, disinformation, hate speech, and other harmful content to be propagated en masse throughout society. It would thus be in the interests of governments and regulatory bodies to work with academic research groups and other entities who are actively developing algorithms and technologies to decipher the language of memes and detect harmful ones.
Funding and support initiatives like the recently announced Centre for Advanced Technologies in Online Safety (CATOS) could expedite their efforts to improve their AI models in order to better account for the multi-modal nature of memes, and to collate together larger and more comprehensive datasets to train their algorithms.
Furthermore, more open lines of communication and exchange of data between research teams and regulatory bodies should be fostered. Research teams may continuously and regularly update government regulatory bodies on the latest forms of harmful memes that have come into circulation and vogue, so that counter-messaging operations may be planned and deployed to stem the negative effects of such memes on their respective societies.
About the Authors
Usman Naseem is Lecturer at the Macquarie University School of Computing, Australia. Asha Hemrajani and Tan E-Reng are Senior Fellow and Research Analyst, respectively, at the Centre of Excellence for National Security (CENS), S. Rajaratnam School of International Studies (RSIS), Nanyang Technological University (NTU), Singapore.