30 June 2022
- RSIS
- Publication
- RSIS Publications
- IP22036 | Machine Learning Predictions on the 20th Politburo Standing Committee of the Communist Party of China
Many scholars and experts have attempted to predict selection outcomes for China’s top leadership by qualitative judgments based on historical and institutional insights. JONGHYUK LEE instead adopts machine learning techniques to quantitatively and systematically evaluate the promotion prospects of China’s political elite. His quantitative model identifies Li Xi, Li Qiang, Chen Min’er, and Cai Qi as powerful contenders for the next leadership, and can indicate the ranking of each candidate, thus showing who among Xi’s allies might have the strongest influence.
COMMENTARY
Elite selection in China has drawn significant attention from people inside and outside of the country given the domestic and international importance of the regime. As the 20th Party Congress (PC) of the Communist Party of China is scheduled to take place later this year, the matter of whom is to be selected to the Politburo Standing Committee in the new leadership has been at the centre of discussion. Yet, current discourse on the issue is mainly based on narrative and qualitative judgments made by identifying General Secretary Xi Jinping’s close confidants without systematic technique. Now, the increasing availability of data sources and computational techniques enables us to run a big-data analysis even on political outcomes in China. In this regard, I adopted a variety of machine learning techniques to quantitatively predict the promotion prospects of incumbent Politburo members to the next Politburo Standing Committee. The data used in this commentary are retrieved from The Database of CCP Elite published by the 21st Century China Center in 2021.
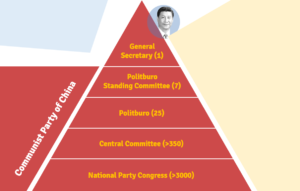
I used predicted probability of promotion as a proxy by which to measure one’s promotion prospects. The predicted probability of promotion allows us to compare the likelihood of a candidate’s promotion to that of others. For the calculation, I employed machine learning predictions where different types of computer algorithms learn historical data patterns and improve prediction accuracy without being explicitly directed to do so. Choosing from among many existing machine learning techniques, I built an ensemble model stacked with six different machine learning algorithms, including linear regressions, penalised linear regressions, random forests, neural nets, gradient boosting machines, and support vector machines. In the analysis, I inserted over 250 features per individual being studied, including those relating to biography (e.g., age, gender, education level, and minority status), career (e.g., number of years of seniority, tenure period, administrative experience, and job diversity), context (e.g., geopolitical conditions prevalent during job tenure), faction (e.g., competing affiliations in top-level Chinese politics), and network (e.g., political connections and centrality indices). Each machine learning algorithm selects critical variables, removes trivial ones, or determines a sufficient variable subset for predictions. Finally, the ensemble model synthesises the results of six models into a single stacked model to maximise the overall prediction accuracy.
The machine learning estimations on predicted probabilities of promotion heavily depend on the scope of data used to train the machine. For the baseline model, I used promotion patterns of the Central Committee members in the 18th PC as a training set to feed the machine for predictions. Two assumptions are made here. The first one is that promotion determinants for Politburo members are similar to those for Central Committee members. Given that the Politburo is a subset of the Central Committee and that Central Committee members are considered a backup pool for the Politburo, applying the same promotion determinants to the Politburo as for the Central Committee seems valid. Second, promotion of current 19th PC members (including those within its subset bodies) will take into account similar considerations that Xi used in the previous PC. It is well documented that Xi has distinctive selection mechanisms (e.g., personal connections) for his high-ranking agents. Thus, in predicting the next leadership, it makes sense to make comparisons against promotion outcomes during only the Xi period.
The accuracy level of the baseline model (after five-fold cross-validations with 1,000 iterations) is around 72 per cent, measured as the area under the curve (AUC) of the receiver operating characteristic (ROC) curve. Scholars generally agree that AUC over 70 per cent is considered acceptable. However, the predictions entirely determined by the machine learning processes tend to ignore the “reality” of the country’s political institutions. For example, there is a rule of “seven up and eight down”, referring to a convention that candidates aged 68 or older are supposed to retire from national-level positions in China. Hence, I will only consider Politburo members whose age is 67 or younger. According to this rule, there are nine Politburo members who have a chance of getting promoted to the Politburo Standing Committee. The rankings of the predicted probabilities of promotion for these nine Politburo members are as follows: Li Xi (29 per cent), Li Qiang (25 per cent), Chen Min’er (12 per cent), Cai Qi (11 per cent), Huang Kunming (6 per cent), Hu Chunhua (4 per cent), Ding Xuexiang (3 per cent), Li Hongzhong (1 per cent), and Chen Quanguo (0.5 per cent).
The result of the machine learning predictions is not surprising at all, given that the top predictions are Xi’s well-known allies. Among nine candidates, six of them have previously been identified as Xi’s followers (i.e., Li Xi, Li Qiang, Chen Min’er, Cai Qi, Huang Kunming, and Ding Xuexiang). It is to some degree expectable that the machine will rank candidates with connections to Xi ahead of those without, because this has been always the main driver of selection during Xi’s administration. One thing distinguishable between previous qualitative analyses and the machine learning quantitative predictions is that we can derive the rankings among Xi’s followers from the latter. Based on anecdotal and historical evidence, we know Xi’s followers would take most of the high-ranking posts. However, the previous discernments could not identify who would likely be second or third in command among Xi’s allies.
The machine learning model predicts that Li Xi is the most likely among Xi’s confidants to be the next Politburo Standing Committee member. However, how Li Xi has the highest probability is unknown since it is a result of an infinite number of interactions between 250 individual variables. It is apparent that the political connection with Xi Jinping is salient, as the list of top predictions includes all of Xi’s known followers. The effect of political connection is heterogeneous, depending on the other features with which the connection interacts. In the analysis, Li Xi is connected with Xi through his earlier postings in Shaanxi. It is well reported that Xi appreciates working experience in his birthplace (i.e., Shaanxi). Other top predictions, such as Li Qiang, Chen Min’er, Cai Qi, and Huang Kunming, are connected with Xi through their working experience in Zhejiang.
The machine learning model also found that the top predictions changed significantly when the model was trained with the promotion patterns during the Jiang Zemin-Hu Jintao period: Hu Chunhua (34 per cent), Huang Kunming (30 per cent), Li Hongzhong (28 per cent), Chen Quanguo (20 per cent), Ding Xuexiang (7 per cent), Li Qiang (5 per cent), Cai Qi (4 per cent), Li Xi (4 per cent), and Chen Min’er (3 per cent). This list may more correctly reflect the promotion mechanisms earlier in the post-Mao period. Certainly, one cannot deny the qualifications of Hu Chunhua. Hu was the youngest member of the 18th Politburo. After completing service with the Communist Youth League, he worked in many poor provinces, including Tibet, Hebei, and Inner Mongolia. Working experience in underdeveloped regions is generally considered important for an official’s promotion. Moreover, prior to Xi’s administration, the cadres nurtured from the Youth League had relatively strong career prospects as a vanguard of communist values with Chinese characteristics, not only as a result of being under Hu Jintao, who himself was from the Youth League, but also under Jiang Zemin. A comparison of the above two predictive lists is evidence that traditional mechanisms such as meritocracy and objective qualifications, esteemed as the most crucial components of China’s remarkable growth, have weakened under Xi’s leadership.
About the Author
Jonghyuk LEE is an Assistant Professor in the China Programme at the Institute of Defence and Strategic Studies, RSIS. His research encompasses a wide range of topics associated with the Chinese political system, including collecting biographical and career data of political elites and predicting career outcomes.
Many scholars and experts have attempted to predict selection outcomes for China’s top leadership by qualitative judgments based on historical and institutional insights. JONGHYUK LEE instead adopts machine learning techniques to quantitatively and systematically evaluate the promotion prospects of China’s political elite. His quantitative model identifies Li Xi, Li Qiang, Chen Min’er, and Cai Qi as powerful contenders for the next leadership, and can indicate the ranking of each candidate, thus showing who among Xi’s allies might have the strongest influence.
COMMENTARY
Elite selection in China has drawn significant attention from people inside and outside of the country given the domestic and international importance of the regime. As the 20th Party Congress (PC) of the Communist Party of China is scheduled to take place later this year, the matter of whom is to be selected to the Politburo Standing Committee in the new leadership has been at the centre of discussion. Yet, current discourse on the issue is mainly based on narrative and qualitative judgments made by identifying General Secretary Xi Jinping’s close confidants without systematic technique. Now, the increasing availability of data sources and computational techniques enables us to run a big-data analysis even on political outcomes in China. In this regard, I adopted a variety of machine learning techniques to quantitatively predict the promotion prospects of incumbent Politburo members to the next Politburo Standing Committee. The data used in this commentary are retrieved from The Database of CCP Elite published by the 21st Century China Center in 2021.
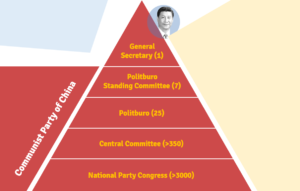
I used predicted probability of promotion as a proxy by which to measure one’s promotion prospects. The predicted probability of promotion allows us to compare the likelihood of a candidate’s promotion to that of others. For the calculation, I employed machine learning predictions where different types of computer algorithms learn historical data patterns and improve prediction accuracy without being explicitly directed to do so. Choosing from among many existing machine learning techniques, I built an ensemble model stacked with six different machine learning algorithms, including linear regressions, penalised linear regressions, random forests, neural nets, gradient boosting machines, and support vector machines. In the analysis, I inserted over 250 features per individual being studied, including those relating to biography (e.g., age, gender, education level, and minority status), career (e.g., number of years of seniority, tenure period, administrative experience, and job diversity), context (e.g., geopolitical conditions prevalent during job tenure), faction (e.g., competing affiliations in top-level Chinese politics), and network (e.g., political connections and centrality indices). Each machine learning algorithm selects critical variables, removes trivial ones, or determines a sufficient variable subset for predictions. Finally, the ensemble model synthesises the results of six models into a single stacked model to maximise the overall prediction accuracy.
The machine learning estimations on predicted probabilities of promotion heavily depend on the scope of data used to train the machine. For the baseline model, I used promotion patterns of the Central Committee members in the 18th PC as a training set to feed the machine for predictions. Two assumptions are made here. The first one is that promotion determinants for Politburo members are similar to those for Central Committee members. Given that the Politburo is a subset of the Central Committee and that Central Committee members are considered a backup pool for the Politburo, applying the same promotion determinants to the Politburo as for the Central Committee seems valid. Second, promotion of current 19th PC members (including those within its subset bodies) will take into account similar considerations that Xi used in the previous PC. It is well documented that Xi has distinctive selection mechanisms (e.g., personal connections) for his high-ranking agents. Thus, in predicting the next leadership, it makes sense to make comparisons against promotion outcomes during only the Xi period.
The accuracy level of the baseline model (after five-fold cross-validations with 1,000 iterations) is around 72 per cent, measured as the area under the curve (AUC) of the receiver operating characteristic (ROC) curve. Scholars generally agree that AUC over 70 per cent is considered acceptable. However, the predictions entirely determined by the machine learning processes tend to ignore the “reality” of the country’s political institutions. For example, there is a rule of “seven up and eight down”, referring to a convention that candidates aged 68 or older are supposed to retire from national-level positions in China. Hence, I will only consider Politburo members whose age is 67 or younger. According to this rule, there are nine Politburo members who have a chance of getting promoted to the Politburo Standing Committee. The rankings of the predicted probabilities of promotion for these nine Politburo members are as follows: Li Xi (29 per cent), Li Qiang (25 per cent), Chen Min’er (12 per cent), Cai Qi (11 per cent), Huang Kunming (6 per cent), Hu Chunhua (4 per cent), Ding Xuexiang (3 per cent), Li Hongzhong (1 per cent), and Chen Quanguo (0.5 per cent).
The result of the machine learning predictions is not surprising at all, given that the top predictions are Xi’s well-known allies. Among nine candidates, six of them have previously been identified as Xi’s followers (i.e., Li Xi, Li Qiang, Chen Min’er, Cai Qi, Huang Kunming, and Ding Xuexiang). It is to some degree expectable that the machine will rank candidates with connections to Xi ahead of those without, because this has been always the main driver of selection during Xi’s administration. One thing distinguishable between previous qualitative analyses and the machine learning quantitative predictions is that we can derive the rankings among Xi’s followers from the latter. Based on anecdotal and historical evidence, we know Xi’s followers would take most of the high-ranking posts. However, the previous discernments could not identify who would likely be second or third in command among Xi’s allies.
The machine learning model predicts that Li Xi is the most likely among Xi’s confidants to be the next Politburo Standing Committee member. However, how Li Xi has the highest probability is unknown since it is a result of an infinite number of interactions between 250 individual variables. It is apparent that the political connection with Xi Jinping is salient, as the list of top predictions includes all of Xi’s known followers. The effect of political connection is heterogeneous, depending on the other features with which the connection interacts. In the analysis, Li Xi is connected with Xi through his earlier postings in Shaanxi. It is well reported that Xi appreciates working experience in his birthplace (i.e., Shaanxi). Other top predictions, such as Li Qiang, Chen Min’er, Cai Qi, and Huang Kunming, are connected with Xi through their working experience in Zhejiang.
The machine learning model also found that the top predictions changed significantly when the model was trained with the promotion patterns during the Jiang Zemin-Hu Jintao period: Hu Chunhua (34 per cent), Huang Kunming (30 per cent), Li Hongzhong (28 per cent), Chen Quanguo (20 per cent), Ding Xuexiang (7 per cent), Li Qiang (5 per cent), Cai Qi (4 per cent), Li Xi (4 per cent), and Chen Min’er (3 per cent). This list may more correctly reflect the promotion mechanisms earlier in the post-Mao period. Certainly, one cannot deny the qualifications of Hu Chunhua. Hu was the youngest member of the 18th Politburo. After completing service with the Communist Youth League, he worked in many poor provinces, including Tibet, Hebei, and Inner Mongolia. Working experience in underdeveloped regions is generally considered important for an official’s promotion. Moreover, prior to Xi’s administration, the cadres nurtured from the Youth League had relatively strong career prospects as a vanguard of communist values with Chinese characteristics, not only as a result of being under Hu Jintao, who himself was from the Youth League, but also under Jiang Zemin. A comparison of the above two predictive lists is evidence that traditional mechanisms such as meritocracy and objective qualifications, esteemed as the most crucial components of China’s remarkable growth, have weakened under Xi’s leadership.
About the Author
Jonghyuk LEE is an Assistant Professor in the China Programme at the Institute of Defence and Strategic Studies, RSIS. His research encompasses a wide range of topics associated with the Chinese political system, including collecting biographical and career data of political elites and predicting career outcomes.